Traditionally, in most B2B enterprises, getting additional revenue from existing customers has a lower cost of sales than getting net new logos. Data from B2B enterprises in 2024 makes it clear that organizations have to increase focus on post-sales.
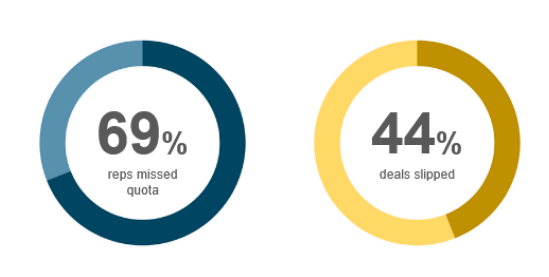
Based on the research from EBSTA over two thirds of sales reps missed quota in 2024 and almost half the deals slipped to subsequent quarters. Things are bleak in post-sales too - since 2022, B2B SaaS has seen NRR decline for the last nine consecutive quarters (Avenir).
Why is revenue signals in post-sales difficult?
Post-sales teams, who are tasked with improving NRR, are being asked to do more with less. Also, in post-sales, revenue opportunities are over a much longer time period compared to pre-sales. POCs and trials are usually a few weeks in duration vs. renewal and expansion deals are spread out over multiple months. POCs and trials are also more tightly controlled compared to post-sales.
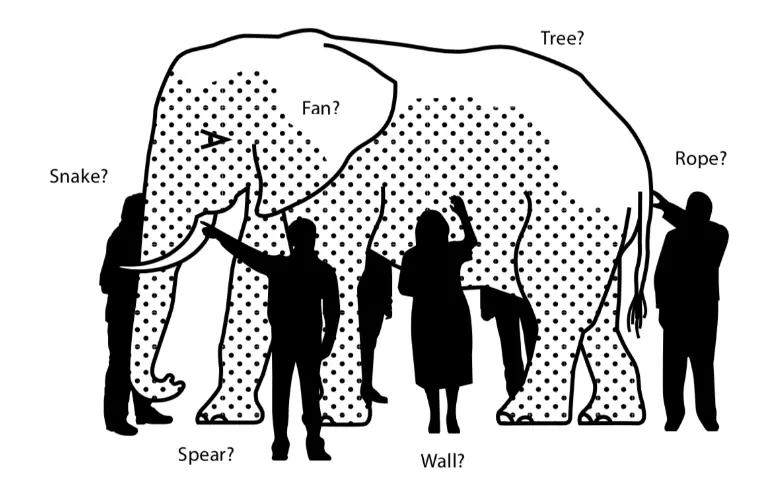
So how do one identify revenue signals in post-sales? This old parable of blind persons and an elephant is an apt analogy here. Different teams in the organization would have a different perspective on how the customer is doing - the customer’s health, their adoption, the customer’s use cases, the value the customer has experienced, etc. Each of the teams will then have a different evaluation of revenue potential. What organizations need is a data-driven approach to revenue signals vs. opinion-based.
How can AI help with revenue signals in post-sales?
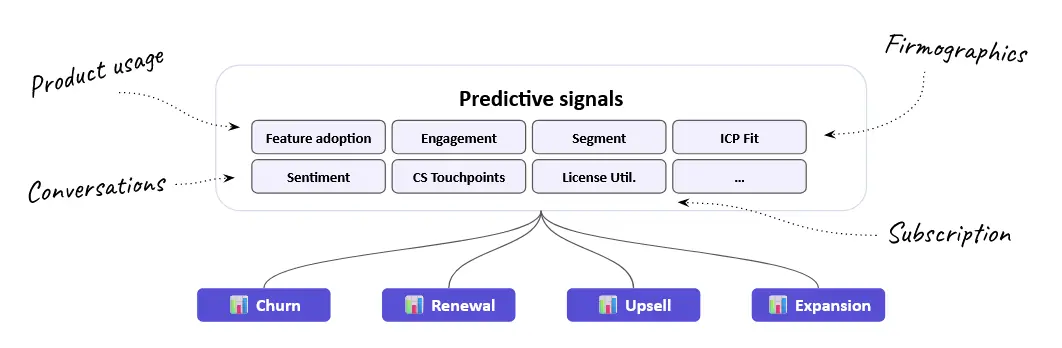
In a data-driven approach, one needs to consider all the various aspects in post-sales. This includes both product and non-product interactions such as product usage, adoption, engagement, support tickets, sentiment, license utilization, etc. One should also consider non-product interactions such as meetings, Slack conversations, etc. that the organization has with the customer. A combination of these data points can give you highly accurate revenue signals. It is often not finding a needle in a haystack; given the amount of data points, it is usually finding a needle in a needlestack. For e.g., a churn risk signal could be from a combination of sentiment change in meetings with the champion and power user, and a steady drop in engagement starting 6 months before renewal date.
AI models, if customized and trained, are ideally suited to do this kind of analysis. The models can be used to identify the “bad” - what factors have contributed to churn in the past. The models can then help identify who among the current customer base has a likelihood of churn and why.
Similar to other use cases, AI is not a one-size-fits-all solution for revenue signals. An important aspect for getting good results is the input data - both in terms of quantity and quality. Another equally important aspect is how good the custom models are. These models need to understand what the data means and then be able to find patterns and use them for signal predictions. With newer and more capable models being released frequently, it is interesting to see what use cases B2B organizations solve using AI!