The paradigm shift brought about by the Product-led Sales (PLS) model has transformed the landscape of the SaaS industry. Unlike the conventional sales-led approach, this model hinges on delivering an outstanding product experience and allowing users to explore it (self-serve) before signing up and paying. Yet, as most conversions occur without direct sales involvement, the challenge emerges: How can one effectively predict revenue with a PLS strategy?
Forecasting within a PLS framework diverges from traditional sales-led methods. To tackle this question, let’s first contrast it with how a sales-led approach functions.
The fundamental difference between sales-led and product-led
Revenue prediction in a sales-led environment involves dealing with a CRM, where selling teams manage deals, track their stages and activities, and utilize the deal pipeline to gauge potential wins. The process often revolves around a simple equation: deal size (a.k.a. average contract value or ACV) multiplied by the likelihood to close (i.e., your win rate probability). However, this process is still largely manual, based on historical data and the intuition of the sales team - which is easily subjected to bias and overconfidence.
Unlike a sales-led scenario where human interaction guides the process, PLS involves numerous self-serve trial signups with minimal sales engagement. Your initial thought might be “Without a sales team, how can we possibly gauge or ascertain a customer's interest in my product?” This thought is natural if you’re new to PLS, but you’d be wrong. There’s even more data at your disposal when leveraging a PLS motion. A better question is, do you have access to the product data from your trial environment that’s needed to forecast? But we’ll revisit this question in a moment.
How are forecasts typically created?
When navigating your transition from sales-led to product-led you might first consider two common forecasting approaches:
Top-Down Approach: This method relies on historical data. It calculates the average conversion rate of your trial leads to paying customers over several months and applies it going forward. This straightforward technique can yield a rough estimate of net new MRR. However, it overlooks variations in conversion rates and the quality of trials, rendering the forecast inconsistent.
Bottom-Up Approach: This approach aligns more closely with traditional sales-led forecasting. It involves evaluating each deal one by one, estimating the likelihood of conversion, and factoring in its potential value. However, unlike the sales-led model, where the likelihood to close is based on the deal stage in the CRM, product-led growth hinges on a different metric.
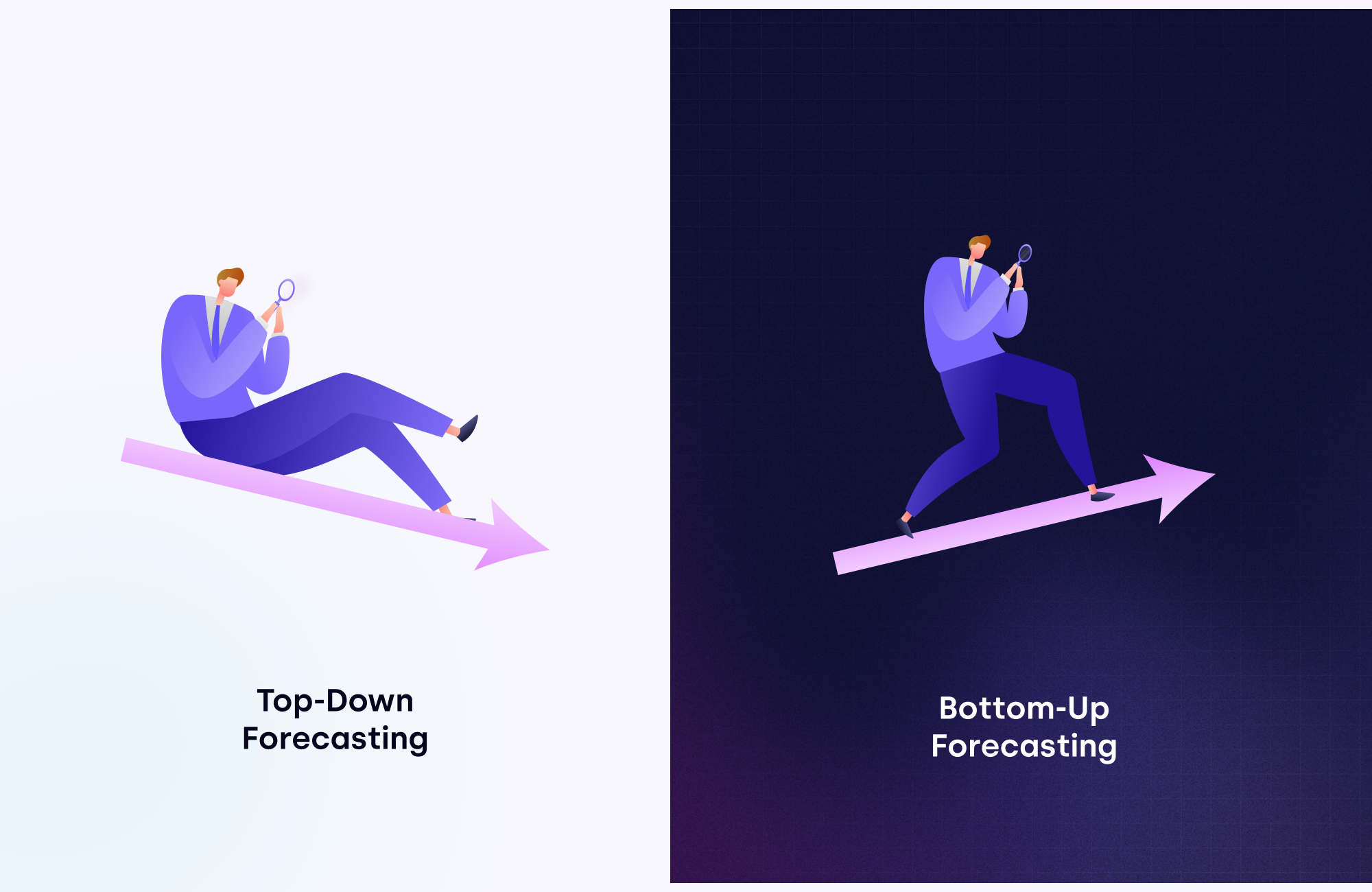
We actually don’t recommend either approach. Instead, you should be basing your PLS forecast on your Activation Rate, which measures the progress a user or account has made toward realizing the full value of your product experience during a trial period. It's a reflection of how far a customer is on their journey to achieving your product's benefits. By examining historical correlations between Activation Rate and conversion likelihood, more accurate forecasts can be generated.
The need for new tooling
In the PLS context, the Activation Rate offers a more objective and precise measure of a user's engagement and potential to convert and therefore introduces new possibilities for understanding user behavior. And by anchoring your revenue forecasts in real user data, accuracy is enhanced. The challenge lies in building a tailored system that suits your organization's unique circumstances.
This brings us back to the other question we posed: Do you have access to the right product data during your product trials? Odds are no, you don’t. Product data is likely buried in your data warehouses and inaccessible to you and your selling teams. Additionally, there are conversational data points taking place around the trial - through Slack, Email, Support Tickets, Product Tickets, etc. - that are also not getting factored into your revenue planning. What you need is a Funnel Intelligence Platform. Tooling that ingests your product and conversational data, allows you to build a customer activity model and then emits real-time revenue intelligence. The classic data, insight, action formula.